This loss is the most commonly used loss is segmentation problems. The IoU-balanced classification loss aims at increasing the gradient of samples with high IoU and decreasing the gradient of samples with low IoU. In this way, the localization accuracy of machine learning models is increased.
Abstract: Image Segmentation has been an active field of research, as it has the potential to fix loopholes in healthcare, and help the mass. In the past years, various papers came up with different objective loss functions used in different cases such as biased data, sparse segmentation, etc. In this paper, we have summarized most of the well-known loss functions widely used in Image segmentation and listed out the cases where their usage can help in fast and better convergence.
In this paper, a novel loss function is present for weakly supervised image segmentation, which, despite its simplicity, performs significantly better than Lagrangian optimization for this task. We achieveclose to full supervision by annotating only a small fraction of the pixels, across three different tasks, and with negligible computation overhead.
While our experiments focused on. In this paper we have summarized such segmentation based loss functions that has been proven to provide state of artin different domains.
These loss function can be widely categorized into categories. Distribution-based, Region-based, Boundary-based, and Compounded (Refer I). Another popular loss function for image segmentation tasks is based on the Dice coefficient, which is essentially a measure of overlap between two samples.

Transfer learning for Segmentation. Each pixel of the output of the network is compared with the corresponding pixel in the ground truth segmentation image.
We apply standard cross-entropy loss on each pixel. We will be using Keras for building and training the segmentation models. About segmentation loss function. I’m doing a project about semantic segmentation.
Since I cannot find a good example for segmentation, my project cannot produce good accuracy. Then, you have the classification using a bounding box regression and the segmentation in parallel. So, you have these three terms that are then combined in a. The soft dice loss is a popular loss function for segmentation models. The advantage of the soft dice loss is that it works well in the presence of imbalanced data.
This is especially important in our task of brain tumor segmentation, when a very small fraction of the brain will be tumor regions. This part of the loss, over here, measures the overlap between.
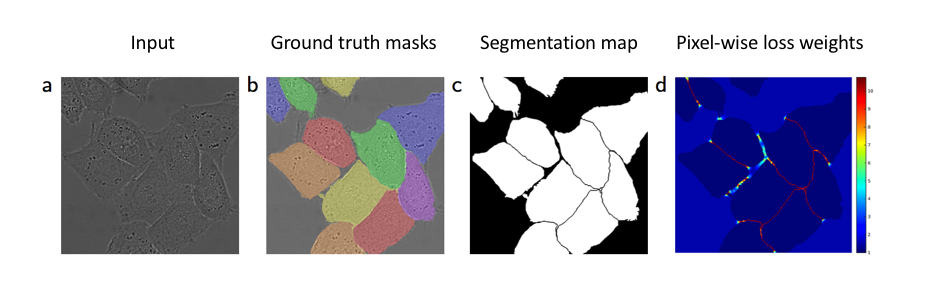
Many loss functions h. Despite the loss of segmentation in various annelid taxa, ontogenetic remnants of their segmented ancestry are present in a number of annelids that do not show obvious segmental features in the adult body (e.g., leeches). Loss of Segmentation. Recent developmental studies have shown that this holds also true for representatives of the Sipuncula (peanut worms), unsegmented lophotrochozoans.
Semantic segmentation is a fundamental problem in computer vision. It is con-sidered as a pixel-wise classification problem in practice, and most segmentation models use a pixel-wise loss as their optimization criterion. However, the pixel-wise loss ignores the dependencies between pixels in an image.
Widely used loss functions for convolutional neural network (CNN) segmentation, e. Dice or cross-entropy, are based on integrals (summations) over the segmentation regions. Les prioriser devient un jeu d’enfant.
Keras image segmentation : loss function for a single pixel. CNN converging only for binary_crossentropy loss function and failing on the test dataset. Hot Network Questions.
It is considered as a pixel-wise classification problem in practice, and most segmentation models use a pixel-wise loss as their optimization criterion. Nevertheless, these methods usually require. It is hard for the network to model the con-textual information from isolated pixels.
Semantic Segmentation is the process of assigning a label to every pixel in the image. This is in stark contrast to classification, where a single label is assigned to the entire picture.
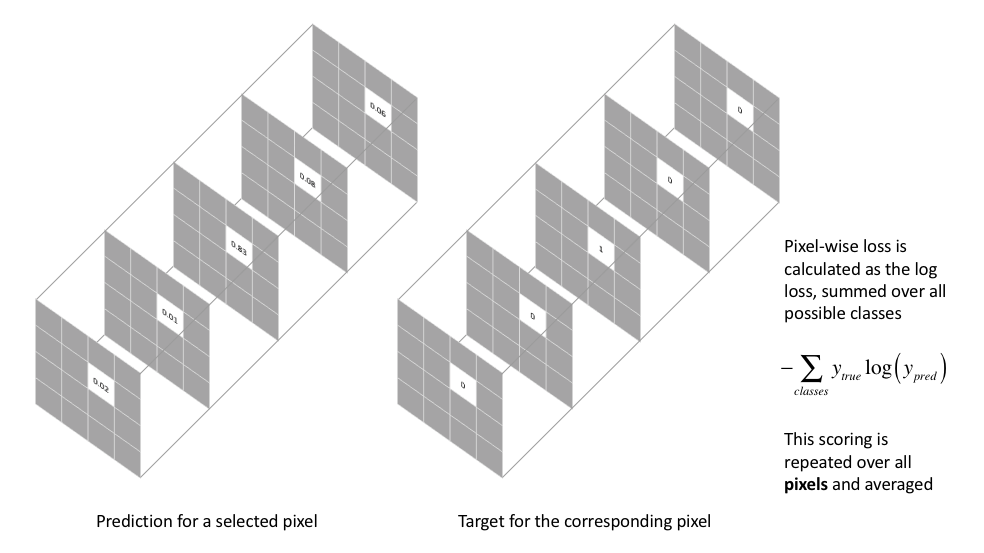
This post is part of the series in which we are going to cover the following topics.
Aucun commentaire:
Enregistrer un commentaire
Remarque : Seul un membre de ce blog est autorisé à enregistrer un commentaire.